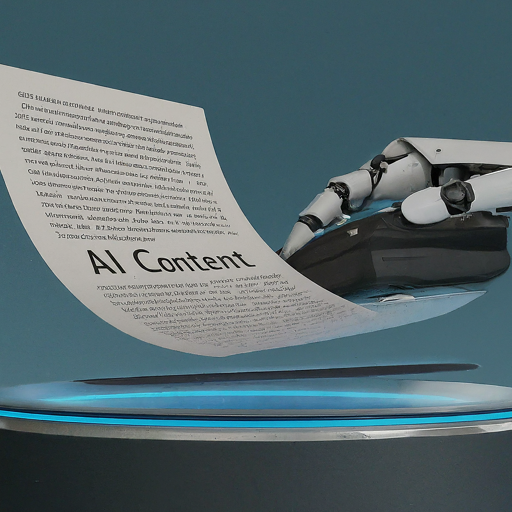
AI Or Human Content? How AI Detectors Are Failing Tests
The lines between human-written and AI-generated text are blurring with alarming speed. As AI language models become more sophisticated, they produce content that’s increasingly difficult to distinguish from the work of a real person. This has spawned the rise of AI content detectors – tools designed to help us ferret out machine-written text amidst a sea of information. Statistics show that about 37.4% of digital marketers are using AI detection tools. However, are these detectors truly up to the task? A growing body of evidence suggests that AI detectors are falling short. Their limitations raise concerns about the reliability of using such software for plagiarism detection, content moderation, and even combating misinformation. Questions emerge: how much can we trust these detectors, and is there a point where the lines will become too blurry even for complex algorithms? Understanding AI Detector Mechanisms AI detectors use unique mechanisms to determine whether the content created is AI-generated. Here are some of the features used: Statistical Pattern Analysis AI detectors dissect writing granularly, seeking predictable word choice and sentence construction patterns. AI language models lean on a narrower vocabulary and may rely on repetitive sentence structures. This predictability stands out to detectors, which calculate statistical metrics to indicate the likelihood that the text is machine-generated rather than coming from a human mind with its unique variations in thought and expression. Plagiarism Detection One mechanism employed by AI detectors involves a vast internal database of existing online content. Incoming text is scanned and compared against this database. If significant similarities or direct matches are found, suspicions arise that the content may not be original. This comparison process is akin to how traditional plagiarism checkers work but is adapted to be more sensitive to the subtle cues that might betray AI-generated writing. Language Model Comparison Source: Originality.AI Another technique involves comparing a piece of text to known outputs from various AI language models. Each AI model leaves a faint statistical “fingerprint” in its generated text. Detectors analyze the writing style, word frequencies, and sentence patterns, attempting to match them against a library of these fingerprints. If a strong match is found, it suggests the text might have been produced by an AI model, not organically by a human writer. Where AI Detectors Fall Short Despite the prominent mechanisms used by AI detectors, there are several shortcomings. They include the following: Evolving AI Models The world of AI language models is in a constant state of rapid evolution. New and improved models are constantly emerging, each more sophisticated than the last. This means AI detectors are in a perpetual race, always playing catch-up. Since they’re often trained on older AI writing samples, they might struggle to identify content from cutting-edge models carefully refined to mimic human writing more convincingly. Limitations in Nuance AI detectors cannot often grasp the subtle nuances present in human language. Sarcasm, irony, humor, and complex layered meanings can easily trip up AI-focused interpretation. Most human readers effortlessly understand these elements, yet they pose a significant roadblock for detectors. This weakness makes these tools less effective in scenarios where context and subtext are essential for understanding the true intent behind a piece of writing. False Positives Source: Positional One of AI detectors’ major shortcomings is their tendency to generate false positives. This means even high-quality, well-written pieces by human authors can be mistakenly flagged as AI-generated. A recent report after testing AI detectors showed that true positive rates varied from 19.8% to 98.4%, which shows how inaccurate they can be. These errors can be caused by a writer’s unusual vocabulary choices, complex sentence structures, or even a writing style that falls outside the statistical norm the detector has been trained on. This issue undermines the reliability of such tools, generating unnecessary worry for human writers. Humans Outsmarting Detectors Humans have mastered the art of tricking AI detectors to score human content. This has brought a major issue when detecting content, Here are some tools that writers use: Paraphrasing Tools Relatively simple paraphrasing tools can surprisingly effectively throw AI detectors off the scent. These tools introduce slight changes to a text by rewording phrases, rearranging sentences, and suggesting synonyms. Even if minor, these alterations can alter the statistical patterns and fingerprints that detectors rely upon, thus making it harder to identify the content as machine-generated. Advanced AI Writing Assistants Tools like Claude.AI represent a new frontier in outsmarting detection algorithms. These sophisticated AI assistants go beyond simple paraphrasing. They focus on generating text that appears organically written by humans, incorporating imperfections and variations that detectors often miss. By actively working to create less statistically predictable content, these AI tools ironically produce results more likely to be mistaken for human work. Intentional Errors In a counter-intuitive twist, deliberate grammatical errors and typos can sometimes be a way to fool AI detectors. Because AI language models are often trained on massive amounts of grammatically correct text, introducing seemingly random mistakes can confuse them. While it’s a risky approach for content meant to be consumed by humans, introducing errors can be a strategy to bypass the digital scrutiny of an AI detector. The Enduring Value of Human Editors Human editors are still the best option when it comes to scoring content and determining if it’s AI written on: Understanding Context Human editors possess an inherent skill that AI detectors struggle to match: understanding context. Language is fluid and carries layered meanings. A human editor can grasp the overall message and intent within writing, ensuring that the subtle nuances and subtext make logical sense. This ability to discern the bigger picture is something machines often struggle with, making human editors critical in interpreting and refining content. Fact-Checking and Verification In an era where misinformation spreads rapidly, the role of a human editor in verifying facts and sources becomes paramount. AI detectors cannot inherently judge the accuracy of information. A human editor can diligently examine claims, research references, and cross-check data to ensure the content is truthfully grounded. This
AI Or Human Content? How AI Detectors Are Failing Tests Read More »